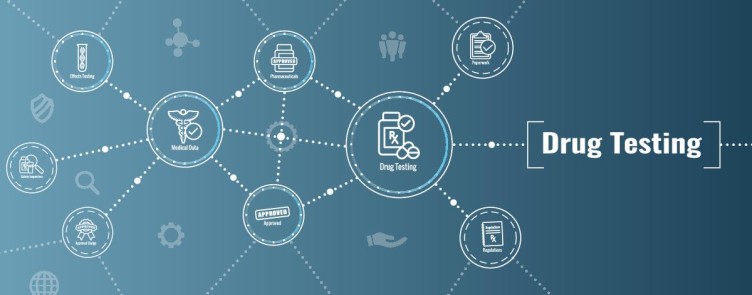
Common Testing Mistakes That Can Impact Your Drug Development Projects Adversely
Stephanie Pasas Farmer, PhD
President and Founder at Ariadne Software
As the drug development process is tightened up, and new processes and guidelines are introduced, the cost of bringing new drugs to market has risen to a soaring $2.558 Billion. Furthermore, only 1 out of 5,000 – 10,000 drugs make it through the entire drug development process, and only 12% of drugs make it through clinical test approvals as per a study conducted by the Tufts Center For The Study of Drug Development in 2014. Although the study is not very recent, it is still very widely accepted. In order to make drug development more efficient, economical, safe and fast, we can do a few things: avoid analysis and testing mistakes, adopt new tools/technologies, and share data from our slip-ups and failed projects so others can avoid wasting time and money on duplicate efforts.
In this article, I will share some common testing mistakes that I have observed in my career as a bioanalyst as well as a consultant and auditor within the industry.
1. Lack of standardization: Even though regulatory guidance documents have been helpful in providing direction toward standardization, there is neither a set format nor a set naming convention to be followed. This makes it very hard to find consistencies from company to company, group to group, and sometimes even from analyst to analyst within the same group.
In fact, I have even observed inconsistencies in naming conventions within the same study document. For example, subject IDs and treatments differ between the “Sample Concentration” and the “Incurred Sample Reanalysis” tables.
2. Propagation of errors due to manual processes: Given the vast amounts of data generated in drug development, it is not uncommon to find an error during manual entries, especially in Method Validation Summary table sections where one needs to pull in data from various sources.
3. Analysis outside of stability period: It is extremely critical to ensure that the analyses are performed within the long-term stability and performed before expiration of critical reagents. However, such errors are pretty commonplace and put the data at risk until long-term stability covering the storage time of the study is proven. Unless the stability is proven after the fact, the data is considered non-reportable or unable to be used, expect for maybe exploratory purposes. Such mistakes can be costly in terms of time delays, the amount of rework required, and sometimes even unusable data.
4. Repeat analysis: When I notice a large percentage of samples requiring repeat analysis within a study, it points toward the weaknesses in the experimental design process. Even in a case where overall repeat analysis is not alarming, it is necessary to evaluate individual analyst performance, such as high failure rates of an analyst during a method validation or sample analysis, or such oversight may result in detrimental data inaccuracies later on.
5. Near-failure/ failure of plate controls: Plate controls are used in method validation and sample analysis to monitor assay performance and to determine if the batch and/or study analysis meets the expected quality standards. Frequently, we see plate controls either trending towards failure or failing during validation and sample analysis without an investigation into a root cause. This calls into question the quality of the data used in validation or study.
It is critical to overcome such challenges to improve the overall efficiency of the drug development process. In order to achieve this, I recommend a 3-step approach:
1. Assess: Within your organization, your department and/or your group, assess what common issues are bioanalysts facing on an everyday basis. For example, an analyst may be utilizing manual data entry processes and generating error-prone data.
2. Analyze: Once you understand the origination of these problems, you can analyze the root cause behind it. In the above example, upon delving deeper you realize that the analyst is inundated with non-standardized data and difficult to read documents, which had been leading to error-prone data. You may even be able to observe patterns in their mistakes during your analysis.
3. Act: Upon learning of the root case, address it and share the learnings with the individual, the larger group and the community alike. In this example, sit with the individual to let them know of their errors and any observed patterns. When possible and/or needed, adopt any tools that may help automate the process to avoid erroneous data and bias that result in costly and time-consuming mistakes. Then share the do’s and don’ts of sample processing within your group, organization and the community.
I hope that sharing of my observations and suggested actions can help you save frustration and otherwise wasted resources that result from such unintentional and unwanted errors and mistakes. As an effort toward raising awareness around sharing lessons learned in drug testing, I would like to hear your observations on some testing mistakes that you have commonly observed and how you have managed to tackle them in the long-term.